Keynote Speakers
Zhongxiang Shen
Professor, Yangtze Delta Region Academy of Beijing Institute of Technology (Jiaxing), ChinaSpeech Title: Overview of Electromagnetic Selective Structures
Abstract: In this talk, we intend to provide a brief overview of electromagnetic selective surfaces/structures (EMSS). According to the properties of an incident electromagnetic wave, EMSS can be divided into four categories: frequency-selective structure (FSS), polarization-selective surface (PSS), angle-selective surface (ASS), and energy-selective surface (ESS). Recent developments and advances in the design of EMSS will also be briefly introduced. Finally, future opportunities in the areas of the design and analysis of EMSS will be suggested.
Biography: Zhongxiang Shen received the B. Eng. degree from the University of Electronic Science and Technology of China, Chengdu, China, in 1987, the M. S. degree from Southeast University, Nanjing, China, in 1990, and the PhD degree from the University of Waterloo, Waterloo, Ontario, Canada, in 1997, all in electrical engineering. From 1990 to 1994, he was with Nanjing University of Aeronautics and Astronautics, China. He was with Com Dev Ltd., Cambridge, Canada, as an Advanced Member of Technical Staff in 1997. He spent six months each in 1998, first with the Gordon McKay Laboratory, Harvard University, Cambridge, MA, and then with the Radiation Laboratory, the University of Michigan, Ann Arbor, MI, as a Postdoctoral Fellow. From Jan. 1999 to December 2023, he was a faculty member (Assistant Professor, Associate Professor and Full Professor) of Nanyang Technological University, Singapore. He is now a Strategic Scientist at Yangtze Delta Region Academy of Beijing Institute of Technology, Jiaxing, Zhejiang, China. Dr. Shen served as the Chair of the IEEE MTT/AP Singapore Chapter in 2009. From Jan. 2010 to Aug. 2014, he was the Chair of IEEE AP-S Chapter Activities Committee. From July 2014 to December 2018, he served as the Secretary of IEEE AP-S. He was an elected AdCom member of the IEEE AP-S from Jan. 2017 to Dec. 2019. He served as an Associate Editor of the IEEE Transactions on Antennas and Propagation from July 2016 to July 2022. From Jan. 2021 to Dec. 2023, he is a Distinguished Lecturer of the IEEE AP-S. Prof. Shen is currently the Editor-in-Chief of IEEE Open Journal of Antennas and Propagation. Prof. Shen is an IEEE Fellow. His research interests include small and planar antennas for various wireless communication systems, analysis and design of frequency-selective structures and absorbers, hybrid numerical techniques for modeling RF/microwave components and antennas. He has authored more than 240 journal papers (among them 180 were published in IEEE Journals) and also presented nearly 200 papers at international conferences.
Qin Zhang
Professor, Institute of Nuclear and New Energy Technology and Department of Computer Science and Technology, Tsinghua University, ChinaSpeech Title: Trustworthy AI Aiding Clinical Diagnosis in Real World
Abstract: Medical AI needs to be trustable. However, the current AI such as Deep Learning and Large Language Models is black-box without explainability. DUCG (Dynamic Uncertain Causality Graph) is a newly developed trustworthy medical AI for clinical diagnosis in general practice and beyond. It graphically represents the medical knowledge and makes probabilistic reasoning with explainability, transparency and inherent invariance in different application scenarios. It is causality-driven instead of data-driven, so that it does not have problems such as data collecting, labeling, training, hallucination, generalization, privacy, bias, high cost and high energy consumption, etc. Cooperated with clinical experts deeply, 46 chief complaint models covering more than 1,500 diseases have been constructed and applied in the real-world in China. 41 models are retrospectively verified by third-party grade IIIA (the highest grade in China) hospitals. The verified diagnostic precisions of the 41 models are no less than 95%, in which the precision for every disease including the uncommon one is no less than 80%. More than 2 million real diagnosis cases have been performed, in which the prospective study was executed to some extent. So far, 17 cases have been identified as incorrect. The mistakes in DUCG were traced and corrected. These mistakes have not been reported since the corrections. Statistics show that DUCG can improve the ability of general practitioners to diagnose diseases several times more than without DUCG. The chief complaints are:
dizziness, headache, nasal congestion (including runny nose), nosebleeds, sore throat (including dry throat, itching, and foreign body sensation), jaundice, swallowing difficulties, cyanosis, coughing and sputum production, difficulty breathing (including chest tightness and shortness of breath), neck and back pain, hemoptysis, lymphadenopathy, chest pain, palpitations, vomiting blood, joint pain, nausea and vomiting, menstrual reduction or amenorrhea, limb numbness, edema, bloody stools, constipation, vulvar itching, abnormal vaginal secretions, vaginal bleeding, rash, fever, anemia, obesity, weight loss, children's fever, abdominal pain, diarrhea, fainting. bloating, oliguria or anuria, lower urinary tract symptoms (frequent urination, urgency, painful urination, gross hematuria, difficulty urinating, polyuria, leakage), lumps A diagnostic model was constructed and tested for more than 70 chief complaint symptoms, including acid reflux, heartburn, belching, ear symptoms (including ear pain, tinnitus, and deafness), scrotal pain, insomnia (including difficulty falling asleep, easy awakening, and early awakening), eye pain, redness, and tearing (including eye pain, redness, tearing, dryness, itching, swelling, and foreign body sensation), visual dysfunction (including visual abnormalities, visual field defects, visual distortion, color vision changes, flash sensation, diplopia, strabismus, and floaters), toothache.
Key Words: Trustworthy, Causality, Diagnosis, Explainability, Probabilistic Reasoning.
Reference:
Zhan Zhang, Qin Zhang, et al. Methodology and real-world applications of dynamic uncertain causality graph for clinical diagnosis with explainability and invariance, Artificial Intelligence Review, (2024) 57:151, DOI: 10.1007/s10462-024-10763-w.
Biography: Qin Zhang graduated from Tsinghua University, Beijing, China, with BS., MS. and Ph.D. Degrees in nuclear engineering in 1982, 1984 and 1989 respectively. He was a visiting scholar with University of Tennessee, Knoxville, TN, USA, and University of California, Los Angeles, CA, USA, from 1987 to1989, working on system reliability engineering and intelligent fault diagnoses. He is a professor of Institute of Nuclear and New Energy Technology and Department of Computer Science and Technology, Tsinghua University, emeritus member of China Association for Science and Technology, member of International Nuclear Energy Academy, fellow of China Association for Artificial Intelligence (CAAI) and director of the specialized committee for causality and uncertainty in AI of CAAI, consultant of the specialized committee for wise medical care of CAAI, Chief scientist of Beijing Yutong Intelligence Technology corp., ltd. He is the former vice president of China Association for Science and Technology, and the standing member of the 13th CPPCC. He originally developed a new AI model called Dynamic Uncertain Causality Graph for fault diagnoses and disease diagnoses. The DUCG medical system has been applied in 4 districts and a county in China for a few years, covering 46 chief complaints such as headache, dizziness, joint pain, etc., including 1500 diseases. More than 2 million cases have been diagnosed with only 17 mistakes identified. All the mistakes were traced and corrected without further mistake reports about them.
Larbi Talbi
Professor, Department of Computer Science and Engineering (DCSE), University of Quebec, CanadaSpeech Title: Improving 6G Network Reliability: Experimental Advances in mmWave Backhaul for NLOS Conditions
Abstract: With the advent of 6G networks, the demand for high-speed, high-capacity wireless communication has never been greater. One of the critical components of 6G infrastructure is the backhaul network, which connects base stations to the core network. Millimeter wave (mmWave) technology, operating in the 30-300 GHz frequency range, offers a promising solution due to its vast available spectrum. However, mmWave signals suffer from high path loss and are significantly attenuated in non-line-of-sight (NLOS) scenarios. This experimental research proposes a new strategy to enhance mmWave backhaul coverage in NLOS conditions, thereby improving the overall performance and reliability of 6G networks. Fig. 1 shows the layout of the scenario and the measurement setup.
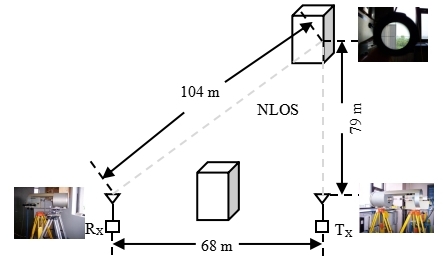
Fig. 1. Schematic diagram of the experimental setup
Biography: Larbi Talbi received the M.S. and Ph.D. degrees in electrical engineering from Laval University, Quebec City, QC, Canada, in 1989 and 1994, respectively. He completed a Postdoctoral Fellowship within the Personal Communications Research Group, INRS-Telecommunications, Montreal, QC, Canada (1994–1995), where he led a project on wireless personal communication supported by Bell-Canada. From 1995 to 1998, he was an Assistant Professor at the Electronics Engineering Department, Riyadh College of Technology, Saudi Arabia. During 1998–1999, he was an Invited Professor at the Electrical and Computer Engineering Department, University Laval, Quebec, Canada. He is currently a Full-Professor at the Department of Computer Science and Engineering (DCSE) at University of Quebec in Outaouais (UQO), Gatineau, QC, Canada, which he joined in 1999. In 2005, he spent his sabbatical leave, as invited researcher, at the Communication Research Center (CRC), Ottawa, Ontario, within the Propagation Research (RVEP) group of the Satellite Communications and Radio Propagation Branch (VPSAT). This collaboration is still ongoing. His research activities include experimental characterization and modeling of millimeter waves indoor radio propagation channels and design of antennas and microwave circuits for wireless communication systems. Presently, he is leading two major projects on designing of new types of metasurfaces for reshaping the propagation channel environments. He has authored and coauthored over 300 journal and conference technical papers. Prof. Talbi is Senior member of IEEE and a member of the Order of Engineers of the Province of Quebec. He regularly acts as a Reviewer for many international scientific journals and conferences and also for research funding organizations.
Fusaomi Nagata
Professor, Department of Mechanical Engineering, Faculty of Engineering, Sanyo-Onoda City University, JapanSpeech Title: Defective Workpiece Sorting Robots Incorporating a Teaching Points Generator and a CNN for Defect Detection
Abstract: What we like to present is two points. One is the automatic teaching point generation for an industrial robot considering undesirable misalignment between robot and work coordinate systems. When a peg-in-hole task with little clearance is tried to be automated by an industrial robot, there is a problem due to the misalignment between robot and work coordinate systems. Such a misalignment, for example, sometimes occurs caused by over and under tightening of screws and bolts used to fix a robot and jigs on a working table, and tends to cause serious troubles such as breakage of workpieces and end-effectors. To cope with the problem, we have developed an application software on Python that automatically generates compensated teaching points for picking and placing only by giving the four corner positions on a working table.
The other is the defective workpiece sorting robot incorporated with a Convolutional Neural Network (CNN) model. Generally, the functionality of the standard teaching interface provided by a robot maker seems to be limited to only the playback-type position control. Also, the extension of the functionality tends to be not easy for users and require much cost. To support the enhancement of the functionality, we have already proposed the Hyper Cutter Location Source (HCLS) data-based robotic interface. The HCLS data interface allows users to build a defective workpiece sorting system by multiple industrial robots incorporated with a CNN model for defect detection. The robots can collaboratively handle a single camera while targeting the sorting of industrial workpieces provided by a manufacturer.
The effectiveness and usefulness of the proposed system implementing the introduced two functions are demonstrated through cooperative peg-in-hole tasks using two small-sized industrial robots MG400s as shown in Fig. 1.
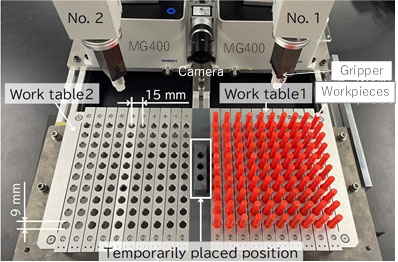
Fig. 1 Experimental setup for peg-in-hole task using two robots MG400s.
Biography: Fusaomi Nagata received the B.E. degree from the Department of Electronic Engineering at Kyushu Institute of Technology in 1985, and the D.E. degree from the Faculty of Engineering Systems and Technology at Saga University in 1999. He was a research engineer with Kyushu Matsushita Electric Co. from 1985 to 1988, and a special researcher with Fukuoka Industrial Technology Centre from 1988 to 2006. He is currently a professor at the Department of Mechanical Engineering, Faculty of Engineering, Tokyo University of Science, Yamaguchi, Japan and also a Dean of the Faculty of Engineering. His research interests include deep convolutional neural networks for visual inspection of resin molded articles, intelligent control for industrial robots and its application to machining process, e.g., robot sander, mold polishing robot, desktop NC machine tool with compliance control capability, machining robot with robotic CAM system, and 3D printer-like data interface for a machining robot have been developed for wood material, aluminum PET bottle mold, LED lens mold, foamed polystyrene, and so on.
Updated soon...