Invited Speaker
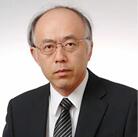
Fusaomi Nagata
Professor, Department of Mechanical Engineering, Faculty of Engineering, Sanyo-Onoda City University, JapanSpeech Title: Defective Workpiece Sorting Robots Incorporating a Teaching Points Generator and a CNN for Defect Detection
Abstract: What we like to present is two points. One is the automatic teaching point generation for an industrial robot considering undesirable misalignment between robot and work coordinate systems. When a peg-in-hole task with little clearance is tried to be automated by an industrial robot, there is a problem due to the misalignment between robot and work coordinate systems. Such a misalignment, for example, sometimes occurs caused by over and under tightening of screws and bolts used to fix a robot and jigs on a working table, and tends to cause serious troubles such as breakage of workpieces and end-effectors. To cope with the problem, we have developed an application software on Python that automatically generates compensated teaching points for picking and placing only by giving the four corner positions on a working table.
The other is the defective workpiece sorting robot incorporated with a Convolutional Neural Network (CNN) model. Generally, the functionality of the standard teaching interface provided by a robot maker seems to be limited to only the playback-type position control. Also, the extension of the functionality tends to be not easy for users and require much cost. To support the enhancement of the functionality, we have already proposed the Hyper Cutter Location Source (HCLS) data-based robotic interface. The HCLS data interface allows users to build a defective workpiece sorting system by multiple industrial robots incorporated with a CNN model for defect detection. The robots can collaboratively handle a single camera while targeting the sorting of industrial workpieces provided by a manufacturer.
The effectiveness and usefulness of the proposed system implementing the introduced two functions are demonstrated through cooperative peg-in-hole tasks using two small-sized industrial robots MG400s as shown in Fig. 1.
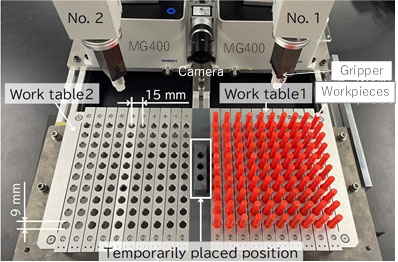
Fig. 1 Experimental setup for peg-in-hole task using two robots MG400s.
Biography: Fusaomi Nagata received the B.E. degree from the Department of Electronic Engineering at Kyushu Institute of Technology in 1985, and the D.E. degree from the Faculty of Engineering Systems and Technology at Saga University in 1999. He was a research engineer with Kyushu Matsushita Electric Co. from 1985 to 1988, and a special researcher with Fukuoka Industrial Technology Centre from 1988 to 2006. He is currently a professor at the Department of Mechanical Engineering, Faculty of Engineering, Tokyo University of Science, Yamaguchi, Japan and also a Dean of the Faculty of Engineering. His research interests include deep convolutional neural networks for visual inspection of resin molded articles, intelligent control for industrial robots and its application to machining process, e.g., robot sander, mold polishing robot, desktop NC machine tool with compliance control capability, machining robot with robotic CAM system, and 3D printer-like data interface for a machining robot have been developed for wood material, aluminum PET bottle mold, LED lens mold, foamed polystyrene, and so on.